Trust & Transparency in Analysis:
- Lisa Ciancarelli
- Apr 8
- 5 min read
Updated: Apr 14
The 5 Essential Practices for Credible Data Sourcing
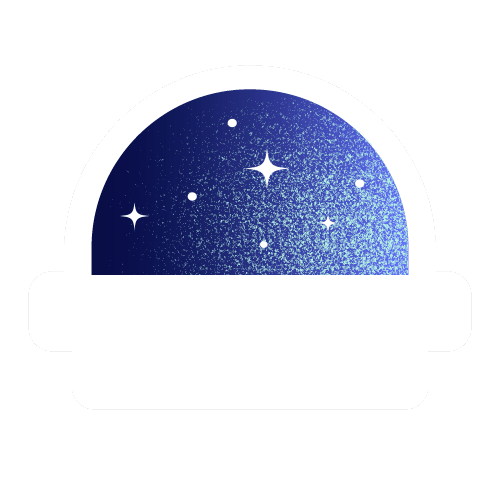
Years ago, I worked for a brilliant researcher who became an incredible mentor to me. He taught me countless lessons about insights and data storytelling, helping shape my career in analytics. He was widely respected in the industry for his knowledge and expertise in data reporting, and I attribute a lot of what I know from the wisdom he shared.
Fast forward several years: I found myself standing in front of this same mentor and his team, presenting data to convince them why their clients should advertise on my company's networks. After I finished my presentation, he looked up at me with that familiar warm smile and said, "You know I love you like one of my own kids, but I'm going to check all of your data!" I smiled back, genuinely delighted at the challenge. "Everything is in the source," I replied confidently. "You can go back and replicate exactly what I did." And so, he and his team did just that!
That moment crystallized something I'd always known to be an indisputable truth: in the world of data storytelling, your insights are only as good as the data on which they stand—AND your willingness to have that data challenged. While compelling visualizations and powerful narratives are expected, your audience's trust hinges on one crucial factor: the credibility of your data sources. Without transparent sourcing practices, even the most visually stunning data story can crumble under scrutiny.
As data professionals, we have an obligation to maintain high standards of quality in what we do. This builds credibility and strengthens the impact of our insights. Today, I'm diving into the five most critical practices for establishing and maintaining credibility through proper use of data sourcing.
1. Embrace Full Transparency in Data Origins
Nothing undermines credibility faster than vague references to data sources. When presenting data insights, be explicit about:
The specific organization or entity that collected or published the data
Time periods covered by the dataset
Sample sizes and population characteristics
Collection methodologies that might influence interpretation
Any known limitations in the dataset
Consider the difference between the two following statements:
❌ "Research shows that remote work increases productivity."
✅ "According to a 2023 Stanford University study tracking 16,000 knowledge workers across 8 industries over 18 months, remote work increased productivity by an average of 13% (with variations by industry), though researchers note limitations in measuring certain collaborative outcomes."
The second approach doesn't just cite a source—it provides context allowing readers to evaluate the relevance and limitations of the findings themselves.
2. Evaluate and Communicate Source Credibility
Not all data sources carry equal weight. A core practice of credible data storytelling is evaluating your sources and transparently communicating why they can be trusted.
When selecting and citing sources, consider:
Methodological rigor — Was the data collected using sound methodological practices?
Reputation — Does the source have a track record of accuracy and integrity?
Independence — Are there potential conflicts of interest that might bias the data?
Peer review — Has the data or methodology been evaluated by independent experts?
Share your evaluation process with your audience. For example: "This analysis draws primarily on data from the Bureau of Labor Statistics, using sampling methodologies and data validation processes. Where possible, we've cross-referenced key findings with data from [note the reports used] to validate."
This approach demonstrates critical thinking and gives your audience confidence that you haven't cherry-picked convenient sources.
3. Disclose Your Methodology
Raw data rarely speaks for itself. The transformations, calculations, and analytical decisions you make shape the story your data tells. Methodological transparency includes:
Data cleaning decisions — What records were excluded and why?
Transformations applied — How did you normalize, aggregate, or otherwise modify the raw data?
Statistical methods used — What specific calculations inform your conclusions?
Tools utilized — What software or analytical frameworks did you employ?
This level of transparency does more than build credibility—it invites meaningful engagement with your work. When readers understand your methodology, they can offer more substantive feedback and engage with your insights more deeply.
4. Address Data Recency and Relevance
Using outdated data without acknowledgment is a common credibility killer. Establish trust by proactively offering:
When the data was collected — Not just publication date, but the actual collection period
Any significant events or changes since collection that might impact interpretation
Why older data might still be relevant (if applicable)
Limitations in applying historical data to current conditions
For instance: "While this survey data from 2022 predates recent regulatory changes, it establishes important baseline measures that help contextualize our more limited current data samples."
By acknowledging temporal context, you demonstrate awareness of data limitations while still offering valuable insights.
5. Confront Conflicting Sources Honestly
Perhaps the greatest test of an analyst's integrity is how to handle contradictory information. When sources conflict:
Acknowledge the discrepancies directly rather than ignoring inconvenient data
Explain methodological differences that might account for varied results
Present multiple perspectives before explaining your analytical choices
Provide rationale for giving more weight to certain sources
For example: "Industry reports suggest 30% growth in this sector, while government data indicates only 15% growth. This discrepancy likely tied to different definitions of sector boundaries, with industry reports including adjacent services. I might chose to use the more conservative government figures as the baseline while noting the definitional differences."
This approach demonstrates intellectual honesty and careful analytical thinking.
🔥Pro Tip - It's always a good final check to review the data citations in your analysis to make sure that you consistently refer to data (don't cite the same point with 2 different numbers!), and confirm it aligns back to your source. This is a solid pre-flight check before sharing your analysis!
Putting all this into Practice
Using these practices doesn't require extensive redesigns of your data storytelling approach. Consider these practical implementations:
Create standardized citation formats for different types of sources
Develop source evaluation rubrics to apply consistently
Include methodology appendices for complex analyses
Design visual cues that quickly communicate source quality
Maintain accessible data documentation that readers can reference
Free Resource: Source Citation Template
To help you implement these practices in your own work, I've created a comprehensive source citation template that you can download for free from my website. This "Source Citation Boilerplate" is especially designed for young professionals and recent graduates who are developing their data storytelling skills.
The template includes:
Standardized formats for citing different types of data sources
Fields for documenting methodology and data limitations
Structured sections for noting data collection periods and sample characteristics
Easy-to-use formatting that can be incorporated into your data spreadsheets
Download this free resource at www.quark-insights.com to start building more credible data stories today.
Conclusion
In an era of information overload and growing skepticism, transparent data sourcing isn't just good practice—it's essential for effective data storytelling. By embracing these five critical practices, you don't just protect your credibility—you enhance the impact of your insights by giving your audience the context they need to truly trust and apply your findings.
Remember, credibility doesn't happen with a single well-sourced analysis but through consistent demonstration of these practices over time. Each transparently sourced data story builds your reputation as a trustworthy guide through complex information landscapes.
